by Antonio Servadio
ABSTRACT
This article delves into the distinctions and connections between big data, data mining, data engineering, artificial intelligence, and Decision Science, aiming to clarify the relationship between these fields. Big data’s value lies not much in its abundance but in transforming its complexity into science-driven decisions. Data engineering plays a vital role in acquiring the right data, while Decision Science fosters a cultural paradigm shift in decision-making processes. The article also questions the notion of “artificial intelligence”, that should rather be considered “assisted intelligence” to aid decision makers. Decision Science empowers decision-makers with mathematical models, digital tools, and algorithms, encouraging critical reasoning over automation. Operations Research emerges as a crucial component in complex decision problem-solving, bridging the gap between mathematics and responsible decision-making. Finally, the relevance of Decision Science in healthcare is highlighted, showcasing its potential impact on personalized medicine and patient-centric care.
I have interviewed Giovanni Righini, professor of Operations Research, who has been developing mathematical optimization algorithms at the Università degli Studi di Milano since 1996. He founded the OptLab laboratory, which over time has established a variety of collaborations with companies and public agencies, carrying out applied research projects. The interview aims to clarify the relationship between big data, artificial intelligence and Decision Science. The latter is an important complement to data science.
DIGITAL DATA AND BIG DATA
Data have always been used as a starting point for solving problems, but the advantage we have today is that if they are available in digital form they can be managed, transferred, queried and processed with high speed and computing power. This makes it possible to solve much more complex problems than in the past and to obtain much more interesting and useful results than could be done in the past. Big data are called this way not only because they are “a lot” but also because they pose new challenges: they are heterogeneous in format (e.g. images, sounds, measurements etc., to be processed together), they are generated continuously, and sometimes need to be processed in real time. Finally, they are not always reliable, because they may contain errors, misses or other flaws. In general, however, what creates value is not data per se but decisions. Data are as useful as oil is: until someone invented the internal combustion engine, oil was just a stinking slurry of little value. If oil is so valuable today, it is because we know how to turn it into energy, that is, into something very useful and versatile. Similarly, big data and all digital data in general gain value when we can turn them into effective, efficient, robust, timely and justifiable decisions. Otherwise, they are just digital garbage – we may call them “big data”, but that does not make them useful.
BIG DATA, DATA MINING, AND DATA ENGINEERING
The Web is an endless source of digital data, and the same can be said locally of the thousands and thousands of information systems and databases scattered everywhere. However, very often the data we need to support good decisions are not “big” and are not found on the Web or in any database; they are the right data, the data we really need in relation to a specific problem we want to solve. To obtain them, one has to observe and measure quantities of interest, and to do so may require designing, organizing and activating an entire process, perhaps complex and expensive, expressly aimed at acquiring that data. Once the necessary data have been obtained, information about the process by which they were obtained is almost as important as the data themselves, as are the instruction manual and the test certificate for a piece of equipment: without a manual, the equipment would be unusable, and without a test certificate, no one would trust it to be used. All this is “data engineering”. Data engineering means considering digital data in the same way as industrial products, which therefore must first be conceived, then designed, produced, tested and certified in order to be sold, used and perhaps even reused or recycled. Such a complex and intricate process means setting up a data tracking system such as it exists for food or pharmaceuticals, for example. To sum up, big data is like a deposit that a mining engineer wants to drill and excavate (it is no coincidence that it is called “data mining”), hoping to extract something valuable from it. Right data, on the other hand, are like products that an industrial engineer wants to produce, purposely setting up an entire plant with related processes. The world is beautiful because it is diverse: there is a lot of work for both mining engineers and industrial production engineers to do. The digital world is also beautiful because it is diverse: there is a lot of work to be done both for those who want to extract value from existing big data and for those who want to engineer the production of right data for specific purposes. There is oil-like data that can only be found in “digital deposits” to be drilled (the big data) hidden amid tons of worthless digital junk, and there is data that can only be produced ad hoc (the right data). Excessive insistence on the importance of big data does not stimulate or help us in engineering the production of right data, neither help the general population to understand how science works.
LEADING TOWARDS DECISION SCIENCE
Transforming decision-making processes through the intelligent use of digital data processed by algorithms is not a trivial step and is not simply the adoption of some new technology: it is a genuine cultural paradigm shift, a different way of thinking, reasoning and decision-making. Therefore, we are beginning to talk about decision science. The fact that a decision is “data-driven” may be a good thing, but it is not when it is meant as an excuse not to exercise that uniquely human endowment that is critical sense, nor is it if it serves the decision maker to shirk responsibility. When the pilot of an airplane goes on autopilot, he still remains awake, alert and responsible for what happens in the air. If in a university examination I establish a grid for grading exercises and a student-A scores 17 thirtieths and a student-B scores 18 thirtieths, before I rule that A is rejected and B is promoted, blindly trusting that “my decision is based on the data”, I should take the trouble to double-check their assignments a second time. And if there are many such cases, the first thing I have to question is not the students’ assignments but my evaluation grid, which proves inadequate to clearly distinguish those who are well prepared from those who are not. In essence, decision science is not about automating decision-making processes, entrusting decisions to algorithms. Instead, it becomes a method of critically reasoning about how decisions are made.
DATA SCIENCE, ARTIFICIAL INTELLIGENCE, AND DECISION-MAKING
To turn digital data into good decisions, there are no shortcuts accompanied by the sign “artificial intelligence”. First, if words still mean anything, artificial intelligence does not exist. What is referred to today as “artificial intelligence” is an extremely heterogeneous set of methods and applications that have in common that they produce some (only some) of the results that humans have produced so far using also (but not only) their own intelligence. This is perhaps a little short to justify the name “artificial intelligence”. To be more precise and respect the meaning of the words, it would be more correct to better distinguish between different systems, such as an automatic control system for autonomous driving, an algorithm for automatic classification of biological samples, and a program for playing chess, instead of calling all three and many other, even more diverse and disparate systems “artificial intelligence.” If it works, it is not “artificial intelligence” but good math and good engineering in the hands of smart decision makers. Without any need to resurrect the philosophical debate on artificial intelligence that was already staged decades ago, it is sufficient to note how unintelligent “artificial intelligence” would be, if it claimed to replace intelligence (without adjectives, because it is the only one). It would be very unintelligent, because the machine does not understand what it is doing and therefore does not exercise critical sense, it cannot think “out of the box.” The machine can solve a given problem well, but it cannot question the definition of the problem it has been given. Thus, it would be better to speak of “assisted intelligence” or “augmented intelligence,” to indicate when intelligence is supported by appropriate artificial tools, making use of algorithms and digital data, which – not surprisingly and not as of today – are called “decision support systems”. But, in this perspective, the roles are reversed: we no longer start from the data, but from the decisions to be made, that is, the decision problems to be solved. It is no longer a matter of data science, but of decision science. The data may not exist yet, but a problem exists and a decision needs to be made. And you want to approach the decision problem with scientific method. How to make effective, efficient, robust, timely and justifiable decisions, making the most of all available tools and knowledge (including digital data, mathematical models, algorithms, hardware devices…)? This is the paradigm shift that needs to be addressed: the shift from decisions made “by intuition” or “by experience” to decisions made by scientific method really deserves the appellation of decision science, because it is something truly new, which cannot be traced back to any pre-existing discipline.
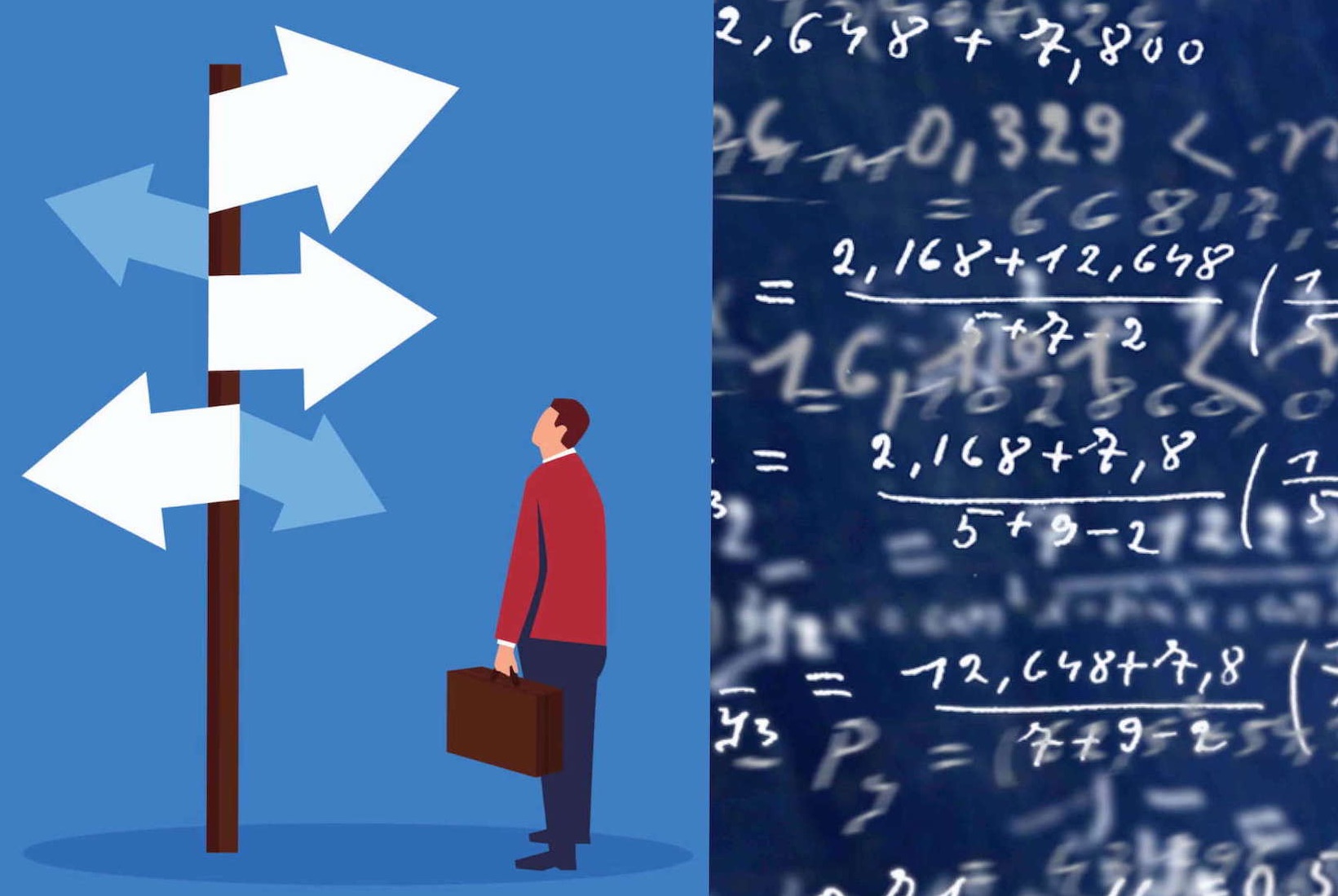
HOW DECISION SCIENCE HELPS US MAKE DECISIONS
Decision science is built around the decision maker, with the express purpose of helping him or her, so that he or she is the one making the decision. The first purpose of decision science is not to suggest a solution, but to provide the decision maker with a tool (mathematical, algorithmic, digital, etc.) to better understand the problem. Of the decision problem, a translation into mathematical terms is given, i.e., a model, and to solve the resulting mathematical problem (typically an optimization problem) one or more algorithms are designed and implemented (and this step requires very specific mathematical optimization skills). However, the use of these algorithms is not immediately aimed at making a decision, but first and foremost at generating solutions that can be useful in questioning the model that generated them. This is how knowledge is produced using the scientific method: by continually questioning a conceptual model, comparing it with experimental evidence or other sources of knowledge that are already available and not necessarily contained in a database (a doctor’s clinical experience, for example, or a political, ethical or value assessment). Only after walking this backward path several times – from “optimal solution” to model and data correction – does one arrive at a model and a data-generation process that are both reliable enough to base good decisions on them. The last step, but only the last, is the assumption of a decision, which at the limit may not even coincide with any of the “optimal solutions” calculated so far by the algorithms. As can be guessed, this way of proceeding is radically different from that criticized, not without some good reason, as “solutionism” (I am thinking of Morozov’s critical positions*).
Decision Science aims to engage decision-makers as active, intelligent, and responsible actors in the use of systems to support their intelligence and decision-making, without shortcuts or automatisms. “Artificial intelligence-based solutions” do not help develop the critical sense essential in the use of data, models and algorithms. On the contrary, experience shows that, not infrequently, when decisions are automated and delegated to algorithms, critical sense (which should distinguish intelligent people) and sense of responsibility (which should distinguish decision-makers) are diminished.
OPERATIONS RESEARCH AND DECISION SCIENCE
For about eighty years there has been a branch of applied mathematics, known in Anglo-Saxon countries as Operations Research / Management Science, which is devoted precisely to the solution by algorithmic means of complex decision problems of various kinds: with one or more decision-makers, with one or more objectives, with certain or uncertain data, etc. Internationally, it is also often referred to as Decision Science. Not surprisingly, the Italian Association of Operations Research (AIRO) has for some years now appropriately re-titled its annual meeting as “Optimization and Decision Science Conference”. Do not be misled by the fact that it is a mathematical discipline – precisely those who are more experienced in algorithmic solution of mathematical optimization problems are also better inoculated against the ideology of “solutionism” and “artificial intelligence” and may realize better than others the need to train new generations of intelligent and responsible decision-makers who know how to use decision support systems with critical sense and competence.
HOW INFORMATICS COME INTO PLAY
Informatics comes into play both as information technology and as computer science, two aspects of the discipline that have been dividing over time. On the technological side, information technology provides techniques and tools without which some applications could not even be thought of. How can one imagine autonomous driving systems without small, light and powerful microprocessors, without digital cameras, without wireless networks, etc.? On the scientific side, computer science continues to study and develop increasingly sophisticated, complex and powerful algorithmic paradigms, such as machine learning algorithms. Optimization algorithms, both exact and approximate, are also an extremely fertile area of research, where the line between operations research and computer science tends to blur.
A QUICK LOOK AT THE RELEVANCE OF DECISION SCIENCE IN HEALTHCARE
Healthcare is one of the most fascinating frontiers for Decision Science, both because of the significance of the expected impact, the delicacy of the decision-making processes involved, and the complexity of the problems to be solved from a technological and algorithmic perspective. In the scientific Operations Research community, many researchers around the world are developing projects in collaboration with hospitals, physicians and health research centers. July of last year, an international conference (ORAHS 2022) was held at the University of Bergamo on this very topic; more than one hundred papers were presented there on as many studies and projects, from therapy optimization to hospital logistics, from emergency management to the definition and simulation of clinical pathways, from planning the use of operating rooms to the analysis of decision-making processes, from optimizing home care services to hospital staff rostering, etc., not to mention all the studies stimulated by the recent pandemic emergency. I would like to add that one of the most important advances expected in the medical field is the development of personalized medicine, that is, the ability to calibrate pharmacological and therapeutic treatments to the specific characteristics of each individual patient. In order to be able to focus the study on the sick person and not only on the disease, it is necessary to focus much more on the use of right data rather than on big data: no patient is an “average patient.”
CONCLUSION
This article aims to clarify differences and links between big data, data mining, data engineering, artificial intelligence, and decision science. By understanding these distinctions, readers can better understand the applications and benefits of each field. All these tools and concepts, when taken together, are crucial to analyze, explore and understand complex systems, enabling science-based decision making.
* Carlo Blengino “Morozov: A Radical Critique of Internet Ideology,” Political Theory. New Annals Series [Online] 6 | 2016, http://journals.openedition.org/tp/710
Antonio Servadio is responsible for Business Development for Technological Innovation at Fondazione UNIMI
Souorce: https://www.linkedin.com/feed/
Pubblicazione gratuita di libera circolazione. Gli Autori non sono soggetti a compensi per le loro opere. Se per errore qualche testo o immagine fosse pubblicato in via inappropriata chiediamo agli Autori di segnalarci il fatto e provvederemo alla sua cancellazione dal sito